Analytics and Student Learning: An Example from InquirySpace
In a pioneering new research direction, the InquirySpace project is capturing real-time changes in students’ development of new knowledge. As students engage in simulation-supported games their actions are automatically logged. By analyzing the logged data, we are able to trace how student knowledge about a simple mechanical system involving a car on a ramp emerges over time.
The ramp game
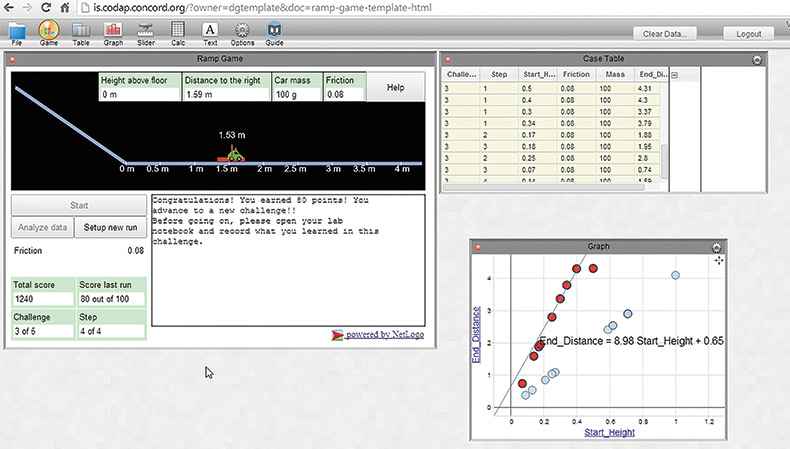
We designed a game for high school students to explore relationships among the variables in a car and ramp system (Figure 1). Students discover how friction, mass and starting height affect the distance a car travels after moving down a ramp. The ramp game consists of five challenges that explore different variables. In each challenge, students must land the car in the center of a target by setting the variables correctly.
After each run, the game provides feedback and a score that serve as incentives for students to use the data from the runs presented in the graph or table to succeed more quickly and accurately. If students come close to or hit the center of the target, they move to the next step within the challenge where the size of the target shrinks. The challenges address different relationships within the ramp system (for example, how friction relates to distance the car travels). Later challenges become more difficult.
Knowledge emergence patterns
We embedded the ramp game in our Common Online Data Analysis Platform (CODAP), so students could collect, select and analyze the data generated during the game. All student actions such as changes to variables in the game (e.g., starting height, mass) and scores are logged automatically in the background. To capture moment-by-moment student learning, we analyzed the log data by applying the enhanced version of the Bayesian Knowledge Tracing (BKT) algorithm used in other intelligent tutoring systems.* The BKT analysis of student scores on each challenge identified seven knowledge emergence patterns shown in Figure 2 and Table 1. After discovering these seven patterns, we used screencast analysis to investigate how well the categorization based on computationally oriented analyses maps onto student learning of new knowledge related to the ramp system.
Screencast analysis
Students in three 9th grade physics classes, two 12th grade honors physics classes and four 11th/12th grade physics classes from two high schools participated in the research, working in groups of two or three. Two groups per class in one school and three groups per class in the other school used screencast software to record their voices and all onscreen actions throughout the ramp game. We used these screencast videos to investigate whether, when and what type of knowledge emerged in each challenge students completed.
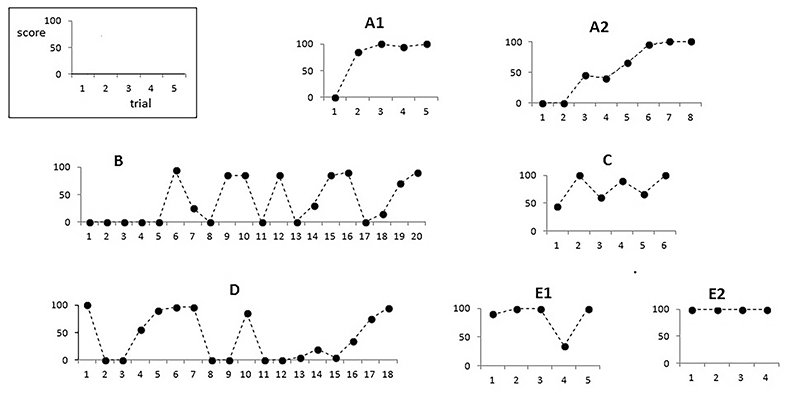
Description | |
---|---|
A1 | Steady and fast mastery of the challenge where scores start low but then quickly become near perfect. |
A2 | Steady but slower mastery of the challenge taking significantly more trials to move from low scores to high scores. |
B | Slow mastery of the challenge after receiving low scores for some time and then changing to high scores with relatively large fluctuations during the change. |
C | Fast mastery of the challenge from medium to high scores with relatively small fluctuations. |
D | Re-mastery of the challenge with relatively large score fluctuations. |
E1 | Near perfect scores, indicating high degree of prior knowledge about the challenge. |
E2 | Perfect scores, indicating students mastered the knowledge prior to the challenge. |
Although the game draws on students’ intuitive knowledge of rolling objects on ramps, the ramp game depends critically on students’ ability to discern patterns in data, abstract the patterns and apply the patterns to new situations in the game. Students develop content knowledge about the ramp system by examining the data in tables and graphs in CODAP. This knowledge includes 1) how height corresponds to end distance for a given friction, 2) how distance changes when friction changes and what that relationship looks like, and 3) whether mass influences the relationship between height and distance.
The ability of students to use the data—their “data knowledge”—ranged from trial and error to more sophisticated strategies where the groups regularly used the table, a point or line on a graph, or used calculators to plug variables into mathematical equations to solve the challenge. We also noticed that students stuck with a particular format such as table, graph or equation, and improved their data knowledge about the preferred format.
Preliminary results from our screencast analysis have revealed that students need both content and data knowledge to succeed. Having only one type of knowledge did not result in accurate predictions. For example, knowing the positive linear relationship between starting height and distance to target is not enough to land the car on the target every time unless students also know how to accurately interpolate a point from the graph.
Combining qualitative and quantitative analyses
Our research on the ramp game shows that 1) knowledge emergence patterns can be identified from the analytics on students’ game scores and 2) these patterns consistently correspond to knowledge emergence events observed in student conversations. By adding the qualitative analysis of the screencasts to quantitative analytics, we were able to uncover more detailed accounts of how knowledge determines student performance on the ramp game.
With real-world validation of student knowledge emergence patterns identified from log data, our next step is to implement these analytics in other instructional games. This is only the beginning, but using real-time data analytics appears to be very promising for automatic diagnostics and real-time scaffolding.
*Corbett, A. & Anderson, J. (1995). Knowledge-tracing: Modeling the acquisition of procedural knowledge. User Modeling and User Adopted Interaction, 4, 253-278.
Amy Pallant (apallant@concord.org) is a senior research scientist.
Hee-Sun Lee (hlee@concord.org) is a research methodologist and science education researcher.
Nathan Kimball (nkimball@concord.org) is a curriculum developer.
This material is based upon work supported by the National Science Foundation under grant IIS-1147621. Any opinions, findings, and conclusions or recommendations expressed in this material are those of the author(s) and do not necessarily reflect the views of the National Science Foundation.