Monday’s Lesson: Using Artificial Intelligence to Design a Solar Farm
A large part of engineering is about searching for good designs. But an optimal design is often hard to find. Artificial Intelligence (AI) is about to change that. Design is an evolution of solutions, and AI tools such as genetic algorithms are an excellent fit. They can generate a variety of designs in the same way genetics does for biology—and help people learn, design, and discover new things. This Monday’s Lesson unveils the incredible power of AI with a classroom-ready application.
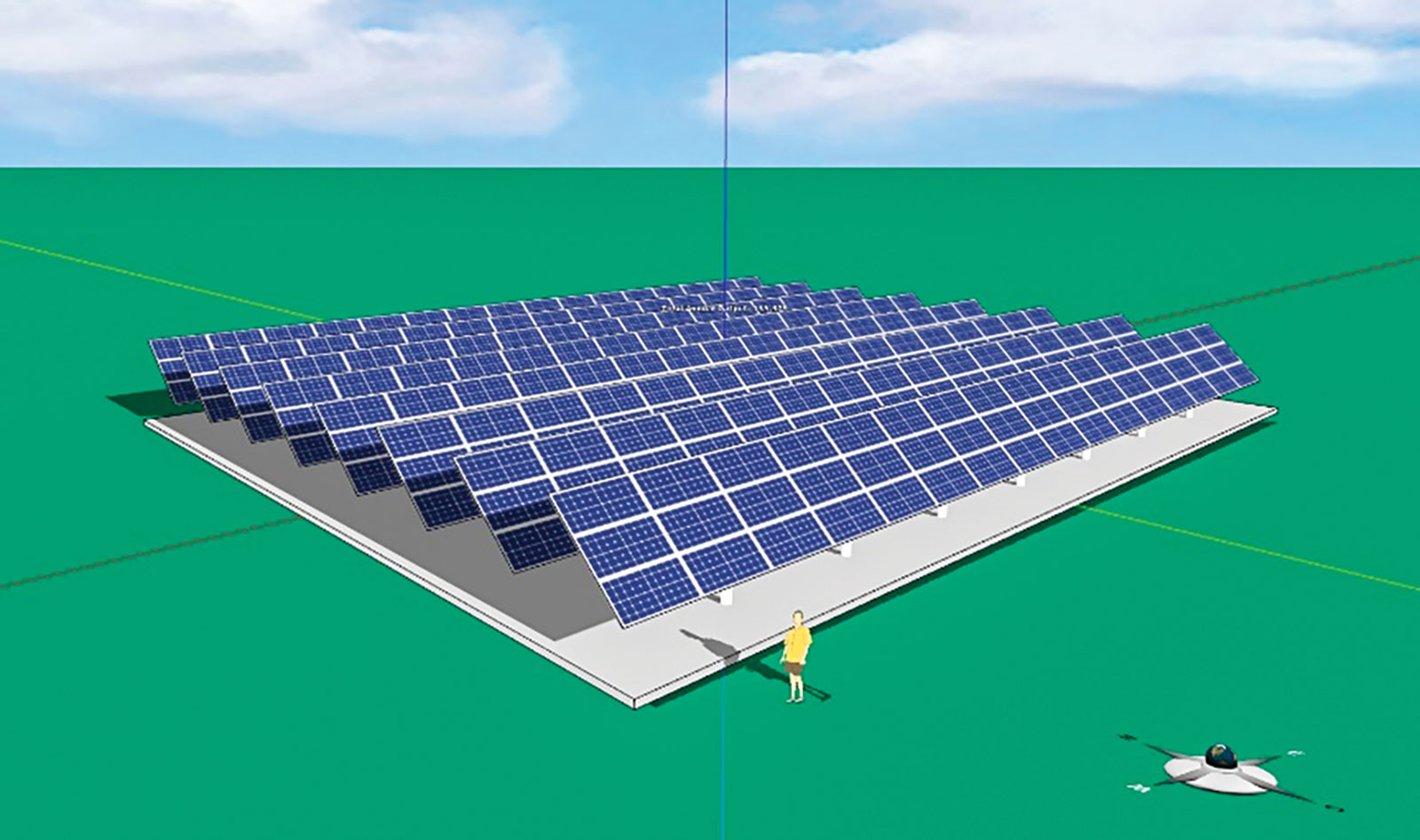
(c)
Designing a solar farm
A solar farm is a power station consisting of photovoltaic panel arrays mounted on the ground. Since it usually involves large capital, engineers must design it carefully to achieve a maximal return on investment. We will use solar farm design as an example to show how students can work with AI to solve complex problems. To begin, download Energy3D.
How does AI work?
AI must know your objective in order to find a solution. For instance, if the objective is to maximize the output without concern for the cost, a design in which the solar panels are closely packed may be a good choice (Figure 1a). If the objective is to maximize the output from each panel, a design with rows of panels far away from one another would be better (unsurprisingly, a single row shown in Figure 1b was found to be the best in this case). If the objective is to maximize the profit, as is often the case in the real world, a design that falls between the two would fit the bill (Figure 1c). You can verify these results with an example provided in Energy3D by opening the menu “Tutorials > Methods of Engineering Design > Solar Farm Optimization.”
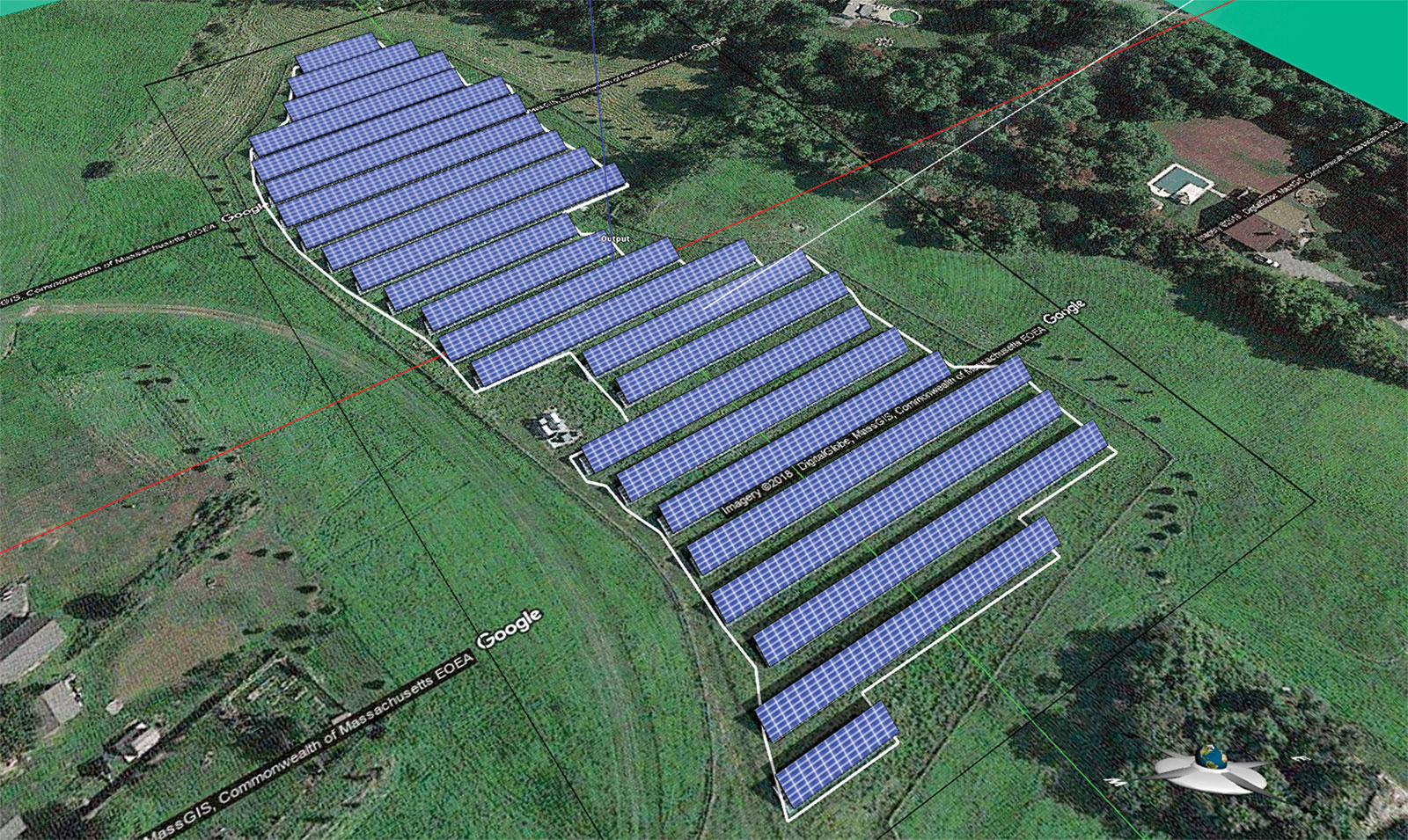
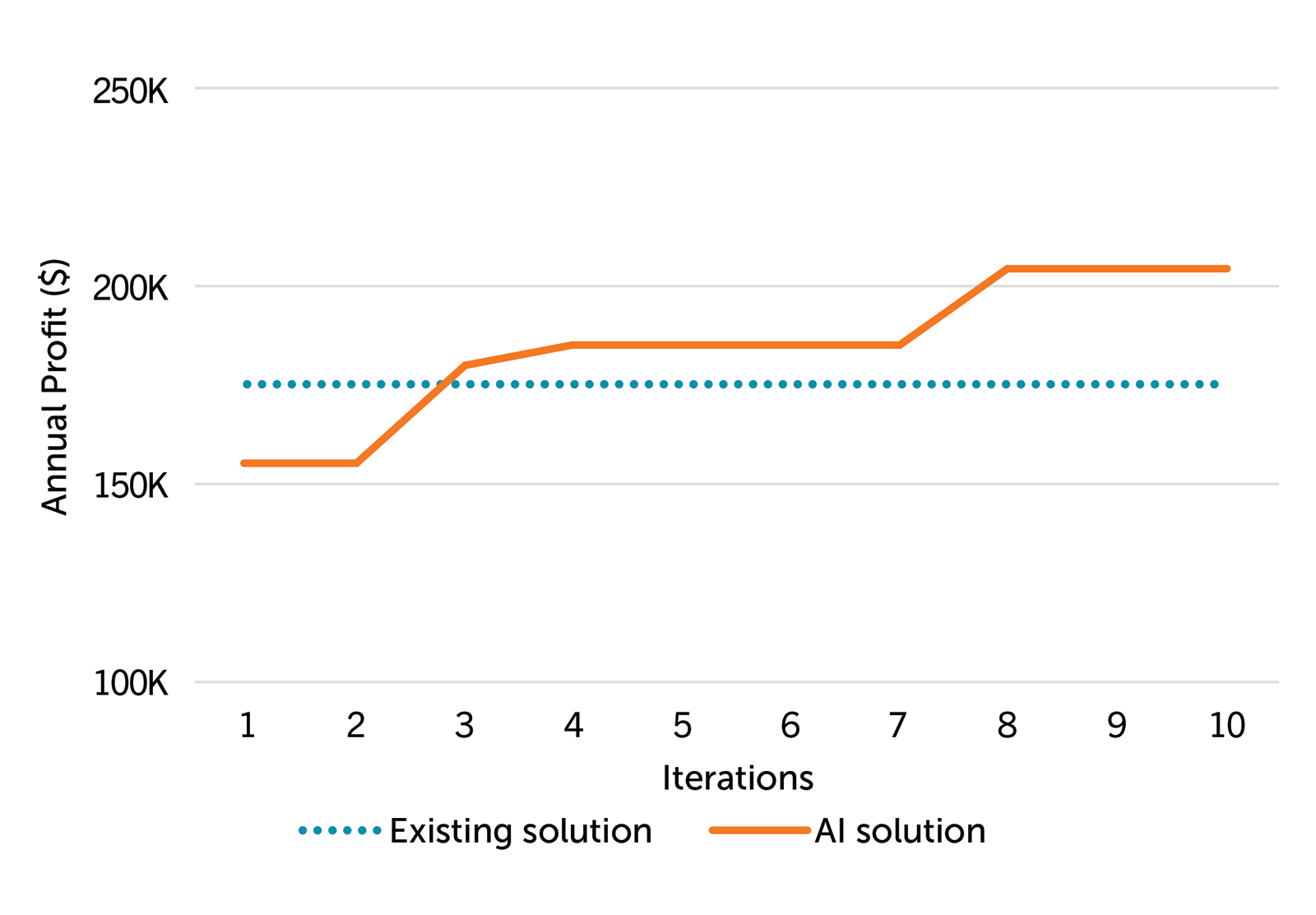
Applying to a real-world project
With Energy3D, you can experiment with a real-world project found through Google Maps. If there is a solar farm in your area, you can easily import its satellite image into Energy3D (using “View > Ground Image > Use Image from Earth View…”) and start modeling it by adding solar panels on top of the image. Alternatively, you can download an existing model on the Virtual Solar Grid as a starting point. Once you have an Energy3D model, draw a minimum polygon that confines all the solar panels on the foundation (Figure 2). This is the area within which AI will search.
Let’s use the solar farm in Figure 2 as an example and show how AI can hypothetically improve it. Figure 3 shows that the AI-generated solutions are inferior to the existing one in the first two iterations, but they surpass it after that. After 10 iterations, AI had evaluated 2,000 solutions (no one can single-handedly do that in the same amount of time) and arrived at a design 16% better than the existing one.
Welcome to the future of design! For the full curriculum module, visit energy.concord.org/energy3d/projects.html
Charles Xie (qxie@concord.org) is a computational scientist.
This material is based upon work supported by the National Science Foundation under grant DRL-1503196. Any opinions, findings, and conclusions or recommendations expressed in this material are those of the author(s) and do not necessarily reflect the views of the National Science Foundation.