K-12 Data Science Education: A Recipe for Success
Data is at the heart of decisions made across all sectors of society, and K-12 education is beginning to take notice. While ensuring that all students learn to work with data fluently is crucial, the path to doing so is unclear, and many open questions exist. Answering them demands cutting-edge research, extensive testing and implementation, and collaboration across many different groups. The Concord Consortium is proud to be at the center of this work with several new initiatives and research projects.
Data science education is a feast of both the familiar and the new, combining traditional statistics instruction and research with intriguing new software tools, data wrangling and management techniques, and interdisciplinary context.
Perfecting any good recipe involves many skills: identifying key ingredients and their optimal ratios, refining the processes of combination, and anticipating ideal conditions. In the field of K-12 data science education, everyone’s still hard at work in the test kitchen assessing essential components—from key competencies to datasets to technology tools and affordances. What pedagogical techniques and curricular treatments can and should be combined? What different educational conditions and settings are needed to pull it all together? We’re seeking answers to these questions and more.
Finding the fundamentals
Datasets are perhaps the central ingredient of data science education. Much more than raw numbers, datasets have order, meaning, and structure. At the heart of any dataset is an object, or “case.” Multiple examples of this case, with one or more associated attributes, comprise a dataset. A lab experiment may capture the state of a system at points in time as its individual cases, with attributes of temperature or position measured for each. Other datasets may choose vastly differing cases—households in a census or individual police stops in a social justice dataset—but the overall “case-value” relationship holds universally.
Although the case-value relationship may seem practically invisible in a two-column table, add one more layer and bigger issues pop into view. What if a lab dataset contains multiple experimental runs? What if a census dataset includes multiple households? Our cases suddenly nest within larger categories. Real-world datasets exhibit such hierarchies all the time, in ways that may shift depending upon the perspective. (Do we group experimental runs by student or control condition? Do we aggregate census households into counties or income bands?) In a new research project, Multidimensional Data, we’re taking a close look at these core components, asking foundational questions about how learners interpret hierarchical data and exploring how novel, technology-based affordances can help deepen understanding and sensemaking (Figure 1).
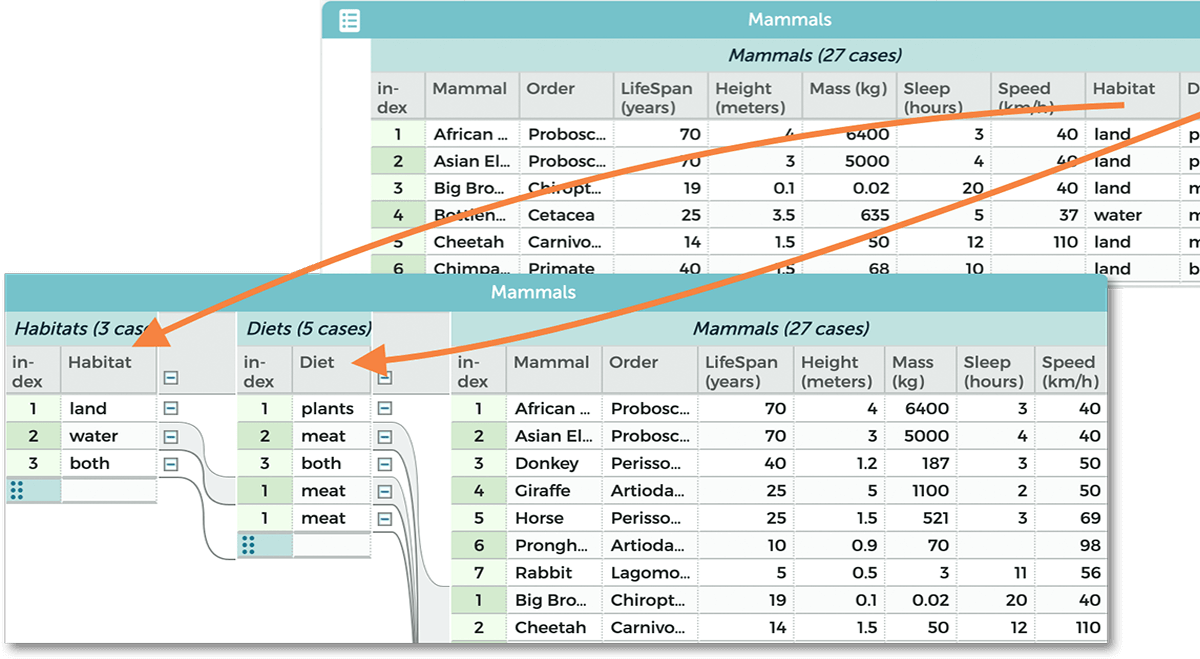
Another question concerns the fundamental types of data, especially one family—data that vary in space and time. From virus tracking to climate change and environmental racism, our understanding hinges on analyzing and tracking patterns in spatiotemporal data. But until the past decade the complexities of doing so have thwarted even science and industry.
Recent advances in computing power and algorithms offer intriguing promise. Yet cognitive and learning sciences still understand little about how learners approach these data or how technology-based tools might help. In our new Data in Space and Time project, we’re joining with leading spatial thinking researchers at Northwestern University and James Madison University to take some of the first steps toward foundational understanding in this crucial new area of study.
These projects offer help navigating ongoing questions about data’s fundamental ingredients. One key lesson we’ve learned: students should understand that data is far more than simply numbers. In our ongoing StoryQ project students learn to view and analyze text and its features as data, demonstrating how data education can help students better engage with the written word—and how doing so can bring data science learning into English classrooms.
Pedagogy and processes
The StoryQ example presages another set of open questions in K-12 data science education, namely where and how we teach about data. These are questions about the processes we use to blend our data ingredients. What does high-quality teaching and learning involving data look like? What experiences are most important? How can we ensure students engage in them effectively?
Some new projects home in on what may be data science education’s largest dilemma. Although many people instinctively place data under the mathematics umbrella, true data science is the interdisciplinary application of data to ask and answer questions within domains. This distinction is critical—data experiences that lack context misrepresent how and why we work with data. Even worse, by hiding data’s relevance to learners’ lives they may turn many, often those already most underrepresented, away from gaining an interest.
Our new DataPBL project is working to correct this bias and investigate how interdisciplinary approaches can help middle school students identify as data-focused learners. By co-designing project-based learning experiences with teachers, in partnership with EL Education, University of Colorado researchers, and UCLA data science curriculum designers, we’re identifying how authentic, high-quality data science education experiences can bridge multiple disciplines.
While data may indeed be everywhere in our world today, very little exists in forms useful for K-12 education. Through initial funding from the Hewlett Foundation, our Open Datasets for Learning project is exploring how datasets can be sourced, prepared, and made broadly available in formats—and with accompanying pedagogical supports— appropriate for use across topics and levels from elementary through high school.
Of course, even the best recipes amount to little in the hands of a chef without sufficient training or experience. In our new ESTEEM II project, we’re building on successful work with North Carolina State University designed to ensure that new teachers become fully prepared to help students gain fluency in working with data. Adopting a systems view, we’re refining and expanding on models developed over years of partnership to develop a network of faculty, organizations, initiatives, and projects focused on transforming undergraduate teacher preparation in data education.
Understanding the conditions
Even the best chefs must be able to work under a variety of conditions. After all, if one is cooking with only an oven, a recipe designed for the stovetop is irrelevant. The difference between formal and informal settings can be equally stark, yet both have promising roles to play in K-12 data science education.
Our new Isles of Ilkmaar project uses the power of games to create a fantasy word with shared experiences in which data plays a critical role (Figure 2). As players—in this project, primarily Latina girls—encounter the game’s scenarios, they come to discover that the key to progress lies in generating, sharing, and analyzing data about its creatures and ecosystems. By purposely making data central to solving problems they find relevant, the game will allow youth to make discoveries together and gain recognition and value for specialized knowledge and skills. By investigating both “game-only” learners and players also involved in after school coding clubs, we will develop a nuanced understanding of how different informal learning settings can interweave to help learners develop identities around reasoning with data.
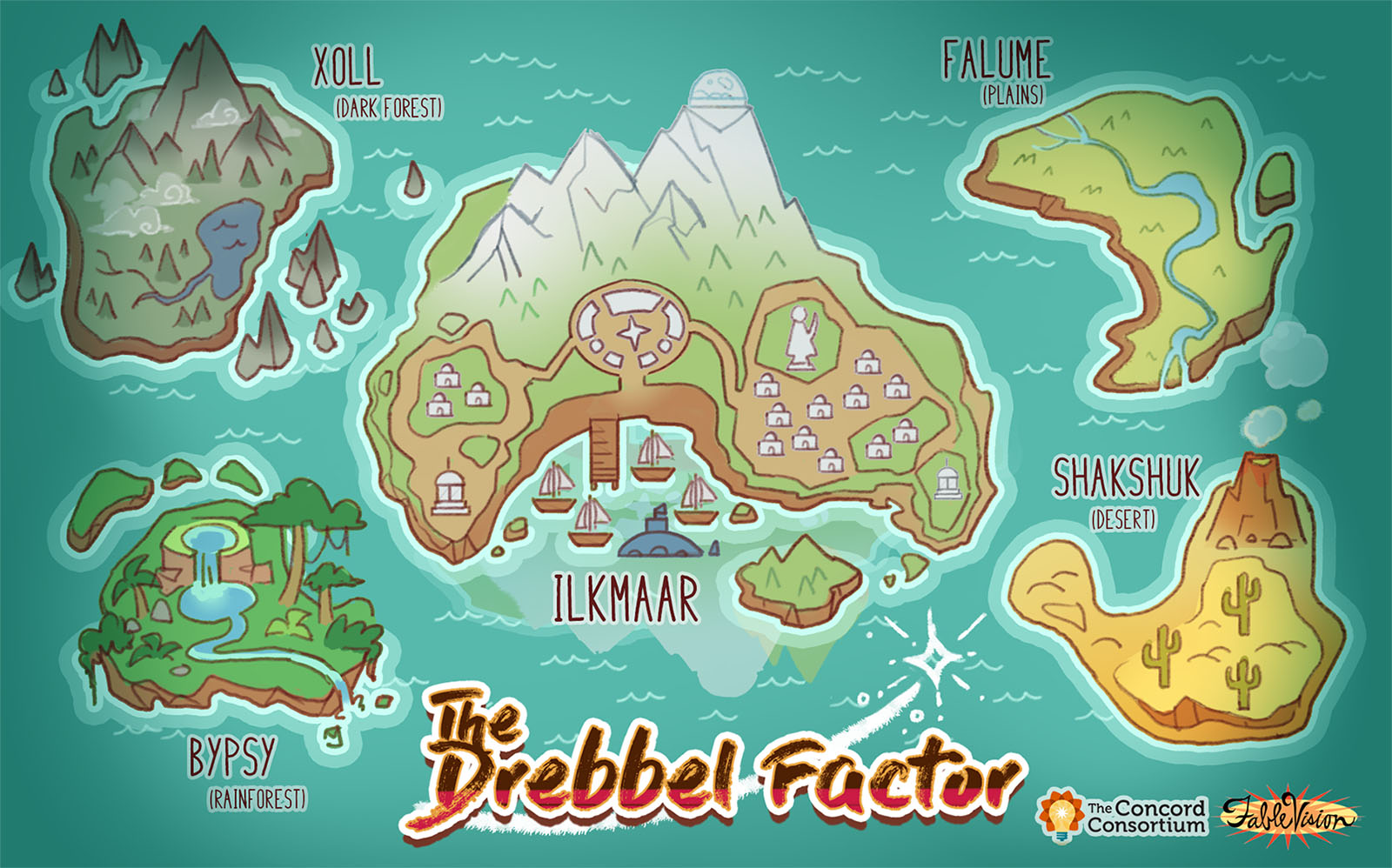
Aiming for the horizon
Together these projects represent a substantial addition to the growing momentum of K-12 data science education research and development. We are proud to add these projects to the extensive body of work we’ve engaged in over many years, including developing tools, convening thought leaders, and building research capacity nationwide. We’re excited to be part of the collective efforts of many data science “chefs” working together to produce a shared result—data science education for all K-12 students.
This material is based upon work supported by the George Lucas Educational Foundation, the Hewlett Foundation, and the National Science Foundation under grant nos. DRL-2201177, DRL-2200887, DRL-2214516, DUE-2201154, and DUE-2141727. Any opinions, findings, and conclusions or recommendations expressed in this material are those of the author(s) and do not necessarily reflect the views of the National Science Foundation.