Teaching Computational Thinking Through Weather Prediction in Alaskan Villages
Research shows that to achieve meaningful learning students should be able to relate what they are learning in school to their real-life experiences. So what happens when students live in a unique environment under circumstances not usually addressed by a conventional curriculum? In Alaska, the distances are vast and many villages can only be reached by boat or plane, not roads. The terrain ranges from expansive forests to treeless tundra and even Arctic “weather deserts” where no snow falls at all. The amount of sunlight also changes drastically throughout the year from long hours of daylight to seemingly endless darkness. Many Native Alaskan villages are unique not only in terms of their geography. They lack services familiar to the lower 48 states: movie theaters, shopping malls, and even road signs. And in some villages people primarily speak Inuktitut or other traditional languages. Developing a curriculum that recognizes and values those differences and relates to the local culture can be empowering for Alaskan Native students and a boost to learning.
Our Precipitating Change project, a multi-year collaboration with Argonne National Laboratory, Millersville University, and the University of Illinois at Chicago, is researching a place-based curriculum in three Native Alaskan villages with a majority of Indigenous students (ranging from 67-95% of the population), where understanding and predicting the weather is a necessary survival skill. We are designing and testing instructional materials and technologies that promote middle school students’ abilities to apply computational thinking practices and understanding in the context of weather and weather prediction.
Because there is little local economic infrastructure in many remote villages, the Native Alaskan way of life is often based on subsistence living: trapping, fishing, and hunting. Success is dependent on understanding upcoming weather conditions and avoiding life-threatening situations such as being stranded in a blizzard. Our objective is to engage students in a relevant and meaningful curriculum that combines real Alaskan data with local culture and knowledge of place.
Learning with Alaskan weather data
Three middle school science teachers from three schools in rural Indigenous Alaska communities implemented a four-week weather unit covering everything from using virtual storm technology and making weather predictions to learning about a career as a weather scientist. Activity and content sequencing were inspired by the practices of professional atmospheric and computational scientists. The curriculum also includes a uniquely Alaskan storyline that ensures alignment among the anchoring phenomena, learning experiences, computational thinking practices, and assessment.
Students assume the role of a local event planner who must decide whether or not weather conditions will allow the Alaskan Native Youth Olympics to proceed as planned. During the lessons, students are introduced to an authentic weather dataset through radar maps and weather station readings (e.g., rainfall, temperature, wind speed, wind direction, and moisture content). They use computational thinking tools and skills (e.g., data abstraction, interpolation, extrapolation, rule abstraction, rule testing, and prediction) to aid them in completing the unit challenge of making an informed weather prediction and presenting their decision. Should the games take place?
The curriculum targets two main Next Generation Science Standards: MS-ESS2-5 (collect data to provide evidence for how the motions and complex interactions of air masses result in changes in weather conditions) and MS-ESS3-2 (analyze and interpret data on natural hazards to forecast future catastrophic events and inform the development of technologies to mitigate their effects). Students and teachers participate in hands-on, inquiry-based activities such as an embedded weather phenomenon in which a virtual storm passes through the classroom. They explore computer modeling and authentic data representations to understand and apply weather-related science and mathematics with data, modeling, and computational thinking skills—the same skills used by real weather scientists. In the final lesson, students can use a digital glossary to learn more about various weather and computational thinking skills terms, and view them as pictures or diagrams or watch a short movie about the context of the words. In addition, students can select Native language words read by Athabascan, Inuit, or Yupik elders to help them understand the vocabulary (Figure 1).
Computational Thinking Skill | Definition | |
Decomposition | ![]() |
Organizing a large amount of information (data) into smaller more manageable parts. |
Pattern Recognition | ![]() |
Recognizing that data or information is repeating in a logical (expected) way. |
Interpolation | ![]() |
Estimating an unknown value based on surrounding information. |
Extrapolation | ![]() |
Following a pattern beyond the known data points. |
Data Aggregation | ![]() |
Collecting and organizing data to better understand the topic. |
Data Abstraction | ![]() |
Simplifying data to make it easier to interpret and find patterns. |
Rule Abstraction | ![]() |
Developing a statement of how things work based on the patterns you observed. |
Model Evaluation | ![]() |
Looking at the big picture by putting all the components together in the model to see if it works. |
Rule Refinement | ![]() |
Modifying a rule to be more precise (exact, careful about details) to work in the model. |
Rule Abstraction | ![]() |
Developing a statement of how things work based on the patterns you observed. |
Research
Research is critical to understand if experiencing real weather events and data enhances students’ science, math, and computational thinking skills and knowledge. We are currently researching the following questions:
- What culturally responsive design adaptations were deemed effective by teachers?
- To what extent is weather an effective STEM subject for increasing middle school students’ computational thinking?
- What are some generalizations that can be made about enhancing students’ computational thinking skills in multiple cultural contexts?
We will assess students’ ability to apply computational thinking skills to solve problems using our Computational Thinking Embedded Assessment Tool, which has been developed and refined by the research team over the previous three years of classroom implementation in Massachusetts, Indiana, and Alaska. Pre- and post-tests will measure changes in students’ computational thinking skills and weather content understanding.
Embedded assessments
Assessments embedded in the curriculum provide opportunities to gauge student understanding without taking time away from instruction. Well-designed embedded assessments seamlessly integrate instruction and assessment. They can provide quality evidence of student knowledge, practice, and development.
The current Precipitating Change curriculum, revised from previous implementations, integrates five learning progression-based embedded assessments designed to elicit students’ developing ideas about computational thinking elements like data abstraction, interpolation, and rule testing. In each assessment, a fictional middle school teacher poses a weather modeling question and four fictional students offer different responses. Each assessment is embedded at both the beginning and end of the relevant unit lesson. On the pre-assessment, students choose the answer they agree with most. On the post-assessment, students discuss the question with peers, choose a response, and then write an individual explanation for why they think their choice is best.
For example, in Lesson 2, students have incomplete weather data for an area and need to interpolate, or estimate a value between known values in surrounding areas. An assessment called How Should We Estimate the Temperature? is embedded in the lesson (Figure 2). The responses from the fictional students are crafted to represent ideas associated with different levels on a computational thinking learning progression. A teacher guide accompanying each embedded assessment provides teachers with short descriptions of theory and background concerning the computational thinking element that is addressed. In addition, the teacher guide suggests ways teachers can scaffold students toward developing more formal understanding (Table 1).
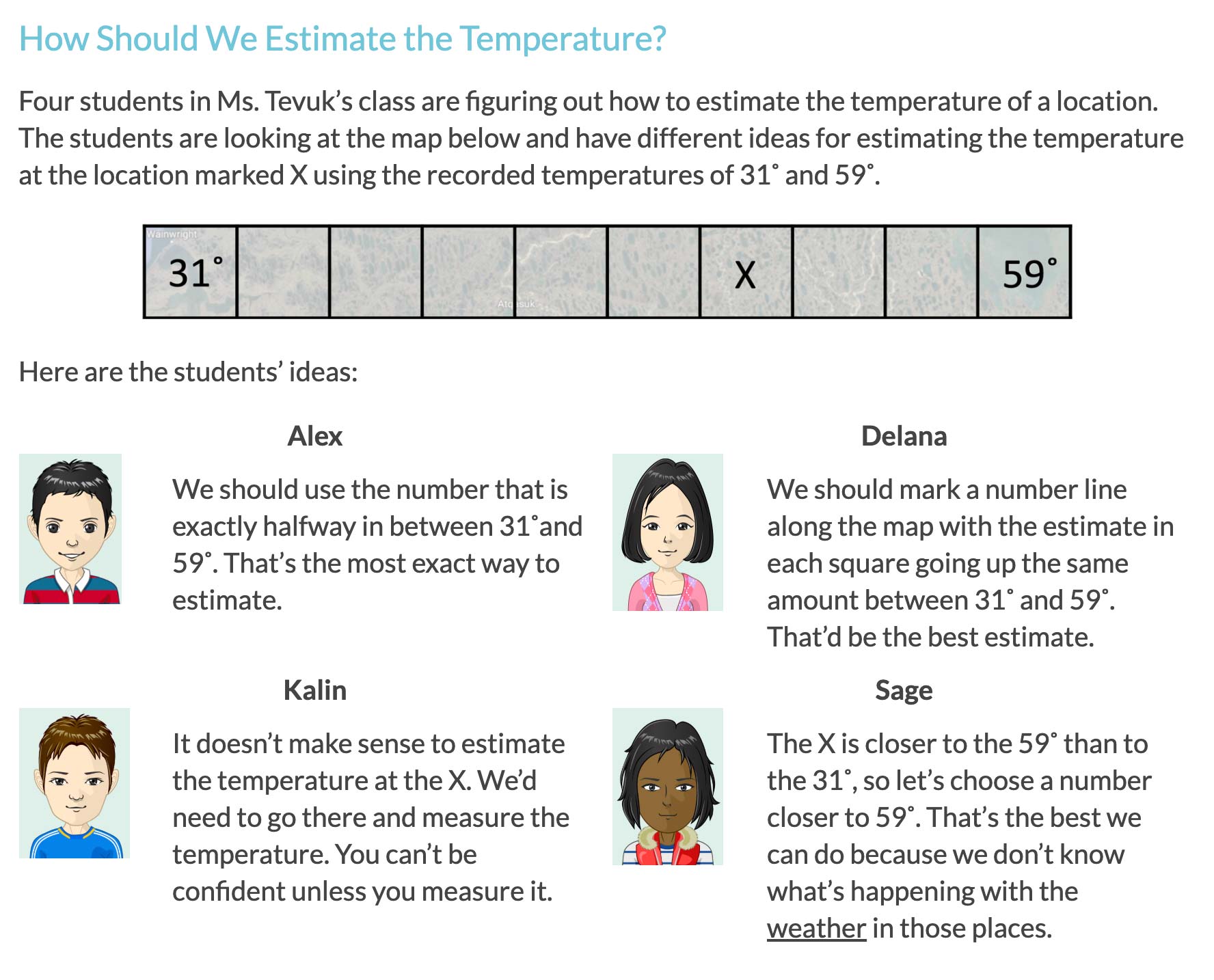
We believe such embedded assessments hold great promise. First, addressing Research Question 1, we’re hopeful that inclusion of optional responses for students provides a safe context for sharing and discussing computational thinking ideas in the classroom. We hypothesize that it is less threatening for students to critique ideas presented by fictional students as opposed to sharing their own original ideas or critiquing their classmates’ ideas. Second, we are optimistic that teachers will find the assessments and teacher guides to be helpful formative assessment tools, especially for those teachers who are new to teaching computational thinking. Finally, addressing Research Question 2, collecting computational thinking data will provide insights into how students are developing and making sense of more formal computational thinking during instruction. Analysis from the embedded assessment implementation will be available soon.
What have we learned?
We plan to conclude our implementation research at our three sites across Alaska in the early spring. Project researchers have spent approximately two weeks at each site working with teachers and observing the rollout of the new curriculum. We were honored to experience the unique cultures of each village, where we were able to learn about teachers’ and students’ lifestyles, as well as how they have adapted to vast distances, isolation, living off the land, and volatile weather.
As curriculum authors, our role is to create 21st century learning experiences that engage students, building on their local customs and ways of learning. Although we have not completed formal analysis of all the data that were collected, we saw firsthand that the integration of a distinctively Alaskan storyline into the curriculum was received well by the students. The annual Alaskan Native Youth Olympics is a widely anticipated event across the state with trophies from competitions of past years adorning schools’ display cabinets.
We also witnessed variation in curricular needs depending on school size. We visited a village with just 11 students in middle school grades (6-8) and another with over 80 students in eighth grade alone. These differences create great challenges for curriculum adaptation, but we continue to work with local teachers to develop creative solutions so that the curriculum can meet their needs.
Throughout the villages, one thing that unites the students is their access to and dependence on the Internet. Although many aspects of Alaskan life are “off the grid,” Alaskans are generally well connected. We hope the Precipitating Change curriculum offers students the chance to expand their computational thinking skills and ultimately contributes in a wide variety of ways to community enrichment.
Student response and reasoning | Scaffolds | |
Less Formal Ideas | Kalin’s response (it doesn’t make sense to estimate; you need to go there and measure) suggests a very concrete thinking approach consistent with the idea that “you’ve got to see it to believe it.” | Students who choose Kalin’s response could benefit from discussions about how while estimates and interpolations may not be perfect, they can still give us a sense of what’s going on in a system when we have limited data. It might be helpful to discuss that we can never collect all the data in the world; there’s too much! Because of this, we need a way to fill in missing values using the data that we do have available. |
Middle Level | Both Alex’s response (choose the value halfway between) and Sage’s response (choose a number closer to 59) might be reasonable estimates in certain circumstances (e.g., for picking out what clothes to wear if you were at location X at that time). These approaches are both better than just choosing a random value as an estimate for X. However, for contexts in which more precision is called for (e.g., making a weather model), then there are ways that we can be more precise in our approaches to interpolation. | Students who choose Alex’s and/or Sage’s response could benefit from discussions of when and why we sometimes might need to be more precise in our estimation approaches. |
More Formal Idea | Delana’s response (mark a number line with the estimate in each square going up the same amount between the two measured values) suggests that she understands which mathematical interpolation approach will give the most precise value. | This level of precision will take more time and effort, and may not even be necessary in some circumstances. However, if our goal is to be precise in our interpolation estimate, then Delana’s response is the most appropriate of the options. |
Carolyn Staudt (cstaudt@concord.org) is a senior scientist.
Texas Gail Raymond (texgail@gmail.com) is a STEM consultant.
Beth Covitt (beth.covitt@umontana.edu) is a science education researcher.
This material is based upon work supported by the National Science Foundation under grant DRL-1640088. Any opinions, findings, and conclusions or recommendations expressed in this material are those of the author(s) and do not necessarily reflect the views of the National Science Foundation.