Can a Robot Help Students Write Better Scientific Arguments?
What if students were able to get immediate feedback on their open-ended responses in science class? Could that dramatically enhance their ability to write scientific arguments? A new project is exploring these questions by investigating the effects of technology-enhanced formative assessments on student construction of scientific arguments.
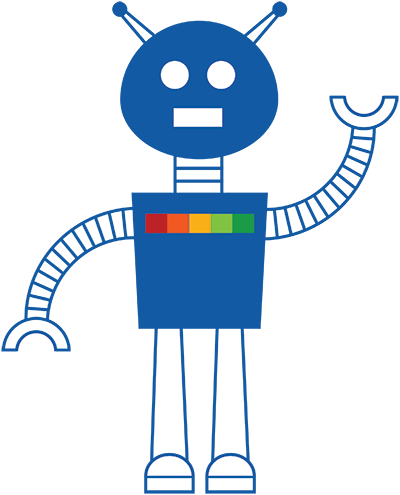
The High-Adventure Science: Automated Scoring for Argumentation project is funded by the National Science Foundation in collaboration with the Concord Consortium and the Educational Testing Service (ETS). HASBot (“HAS” from the High-Adventure Science Earth science curriculum and “Bot” from robot for the automated nature of the feedback) uses an automated scoring engine to assess students’ written responses in real time and provide immediate feedback (Figure 1).
HASBot is powered by the ETS automated scoring engine, c-rater-ML, which uses natural language processing techniques to score the content of students’ written arguments. The c-rater-ML platform was integrated into two High- Adventure Science curriculum modules, “What is the future of Earth’s climate?” and “Will there be enough fresh water?” In each module, students encounter eight scientific argumentation tasks, in which they use evidence from models and data to construct scientific arguments.
Each argumentation task is designed as a four-part item set, including: 1) a multiple-choice claim, 2) an open-ended explanation, 3) a certainty rating on a five-point Likert scale (from very uncertain to very certain), and 4) an open-ended rationale for the certainty rating. Previously, students would have to wait until their teacher had read their responses to get feedback.
Students now get just-in-time feedback that encourages additional experiments with the models, a closer look at the data, and the opportunity to add more evidence and reasoning to their explanations. The goal of the feedback is to help students build stronger scientific arguments.
Preparing for launch
Automated scoring is based on rubrics that differentiate student responses into five categories for explanations and five for certainty rationale, and includes feedback that is specific for each category (Table 1). Developing automated scoring models for the open-ended questions requires a large number of human-scored student responses. Fortunately, we have thousands of such human-scored responses from years of HAS implementations of the climate and water modules. Two project staff members scored these student responses independently. The reliability between the two human coders was excellent, ranging from 0.82 to 0.95 in kappa (k). Kappa is a statistic that represents inter-rater agreement ranging from -1 (less than chance agreement) to 1 (exact agreement).
The next step was to divide the human-scored data into two parts: a training set and a testing set. Approximately two-thirds of the data were used to train c-rater-ML, which generated a scoring model for each open-ended response argumentation task. The remaining data were then used to evaluate the computer-generated scoring model.
When a test set of responses was run through the c-rater-ML models from the training set, human-machine score agreement was between 0.70 and 0.89 k, which is an acceptable threshold for instructional purposes. In half of the items, however, the human-machine agreement was more than 0.10 k lower than the human-human agreement.
While it is not surprising that human-human agreement was higher than human-machine agreement, we were pleased with these initial results. We hypothesize that a lack of student responses on the higher end of the rubric may explain some of this variability, and that as student arguments improve with the use of HASBot’s formative feedback, we will be able to retrain the c-rater-ML models with a wider range of responses and improve the accuracy of the automated scoring.
HASBot’s mission
The four-part argumentation item sets were organized into a block that requires students to answer all four items before submitting their responses for scoring. C-rater-ML then analyzes students’ written responses to the explanation and certainty rationale and returns numerical scores. After students submit their answers, the HASBot robot appears, prompting students to review their scores, displayed on a rainbow bar along with feedback, and inviting students to revise their answers (Figure 2).
We piloted the color score and feedback features in the spring of 2015 and were curious whether students would embrace, reject, or question the validity of the automated feedback. Students liked receiving feedback from HASBot because it was instantaneous and allowed them the opportunity to improve their answers. As one student aptly noted, “robots are non-judgmental.”
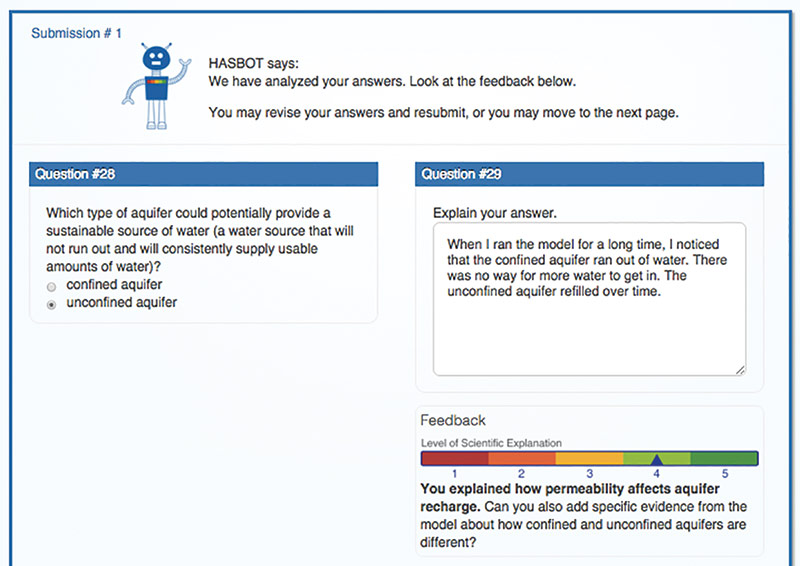
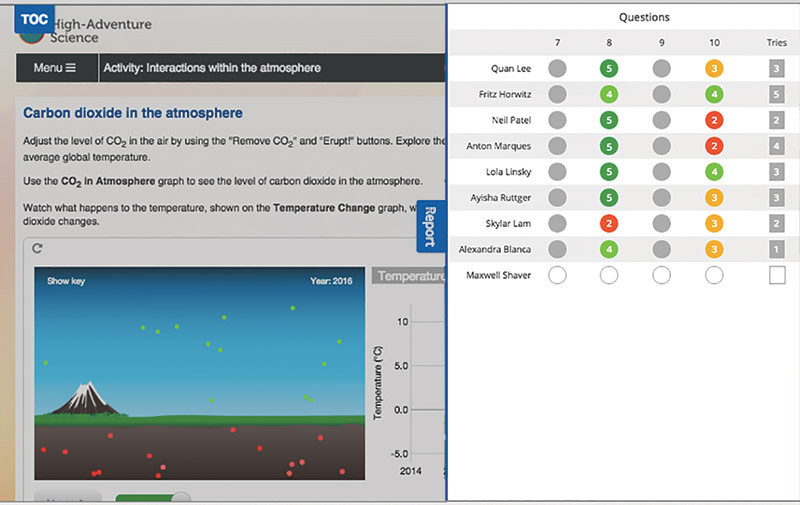
In the first year of classroom implementation, students have generally responded positively to the feedback. Log files and student screencasts (in which we record student actions on the computer as well as their voices) reveal that students frequently revised their arguments based on HASBot’s feedback, some of them many times. One teacher reported that her students “were surprised to get a score of 2 or 3. So it was a bit humbling for students, but they edited their answer and were determined to get a higher score. Success!”
One student said that automated feedback “teaches me to pay close attention to detail and how to correctly fix my mistakes.” Another felt the feedback would help him “become very good at answering questions with justified reasoning.” However, several students wanted more specific feedback with details on their mistakes and explicit hints to improve their answers. We are currently creating and testing contextualized feedback to point students towards more specific ways to improve their arguments. We will test this feature in classrooms in spring 2016, and further refine our design based on the results of each classroom study.
Navigating into the unknown
In our quest to help students improve their scientific arguments, we have not abandoned teachers. They must also navigate the new world of automated scoring. We have developed a dashboard to help teachers keep track of student progress in real time. Before HASBot, teacher reports were limited to viewing student responses after class in a report separated from the activity. With the new dashboard, teachers can see their students’ responses to the argumentation items in real time while students are still in the classroom (as well as after class).
A table of contents tab shows the location of students in the activity. On pages with an argumentation item set, a report tab shows student scores for each open-ended question using the same rainbow color scheme students see (Figure 3). Teachers can drill down into the report to see each individual student’s responses to the argumentation item sets or an aggregated view of all responses to a particular item. We will pilot the new teacher dashboard in a small number of classrooms this year.
Score | Meaning | General feedback to student |
---|---|---|
1 | Off-task or non-scientific answer | You haven’t explained your claim. Look again at the pictures/models. |
2 | Student repeated claim | You made a claim without an explanation. |
3 | Student made associations | Your explanation needs more details. |
4 | Student used data or reasoning | You used evidence from the pictures/model or explained why this phenomenon happens. |
5 | Student used data and reasoning | You used evidence and reasoning. |
Table 1. Detailed scoring rubrics differentiate student responses into five categories for explanations and assign a color code to each score.
Looking toward the future
We will refine c-rater-ML scoring models, HASBot feedback, and the teacher dashboard based on data from ongoing classroom implementations. Reactions by both teachers and students have been promising and we are cautiously optimistic that automated scoring and feedback can play a central role in helping students develop the practice of scientific argumentation. With HASBot as our co-pilot on this journey, our goal is to discover the best ways to use enhanced technological tools to improve science learning.
Trudi Lord (tlord@concord.org) is a project manager.
Amy Pallant (apallant@concord.org) directs the High-Adventure Science projects.
This material is based upon work supported by the National Science Foundation under grant DRL-1418019. Any opinions, findings, and conclusions or recommendations expressed in this material are those of the author(s) and do not necessarily reflect the views of the National Science Foundation.