Data Science Games
The emerging discipline of data science combines computational thinking, mathematics, statistics, and content knowledge, paving the way for a new genre of educational technology: data science games. Funded by the National Science Foundation, our Data Science Games project is developing games and curriculum materials for middle and high school students to use data while learning science. The goal is to research the potential of this new genre of educational technology.
Data science games
The more experience students have working with data, the better prepared they are to contribute to the data-driven society they are entering. Students practice data gathering and interpretation best in the context of learning subject-specific material. But middle and high school students currently do not get much experience working with data. Our Data Science Games project is exploring how games about science that include data at their core can be integrated into classroom learning in schools that have adopted Next Generation Science Standards (NGSS).
All data science games are embedded in our Common Online Data Analysis Platform (CODAP), so students can analyze the data generated in each game. The games follow a similar design: students generate data through their actions and make sense of that data as essential moves for game play. CODAP allows students to store, organize, analyze, and visualize their data. By combining, filtering, and transforming the data, students better understand the game, improve their game strategy, and level up—plus experience data science at the same time.
Games for physics, chemistry, and biology
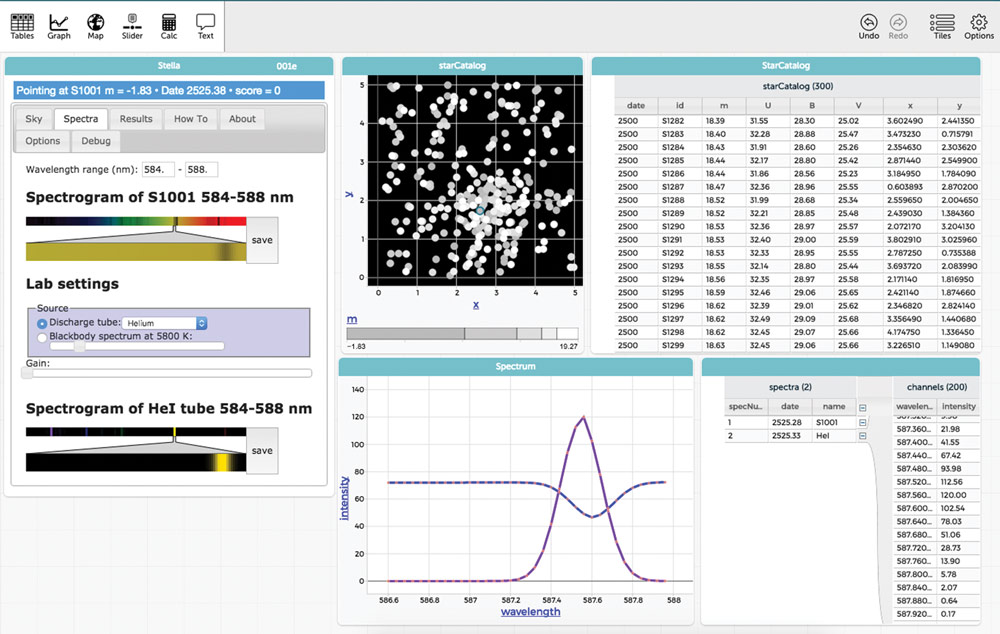
In the game Stella the goal is to find information about a star, such as the speed with which it’s receding from the Earth (Figure 1). In this simulation, students compare the color spectrum of a star with elemental spectra to detect the pattern of its chemical composition. The score depends on the ability to use data about spectral lines to find the star’s “red shift.” Stella helps students build an understanding of the NGSS HS-PS3-3 performance expectation, so they learn science content in the context of a game.
We are also exploring additional games for physics, chemistry, and biology (Figure 2). For example, another physics data science game might involve building or altering a structure within certain constraints; the data include the forces on all the structural elements. A chemistry game might incorporate the custom design of chemical reactions to achieve specific goals, such as buffering a chemical system so it doesn’t explode. Each reaction could generate data about bond strength, activation energy, pressure, temperature, and concentration that students use to solve specific problems or puzzles.
And a biology game could consist of an epidemiology puzzle, in which the goal is to stop the spread of a highly contagious disease. For each move, students are allowed to examine some of the patients who come to the clinic for treatment. Students can use maps in CODAP to visualize data about the spread of disease among clinic patients in the same neighborhood or workplace. The score is determined by how quickly the student stops the spread of disease.
We are currently developing and testing beta versions of these games to ensure they are engaging and educational. Future versions of the games will be released under open content licensing and available at no cost.
Classroom testing
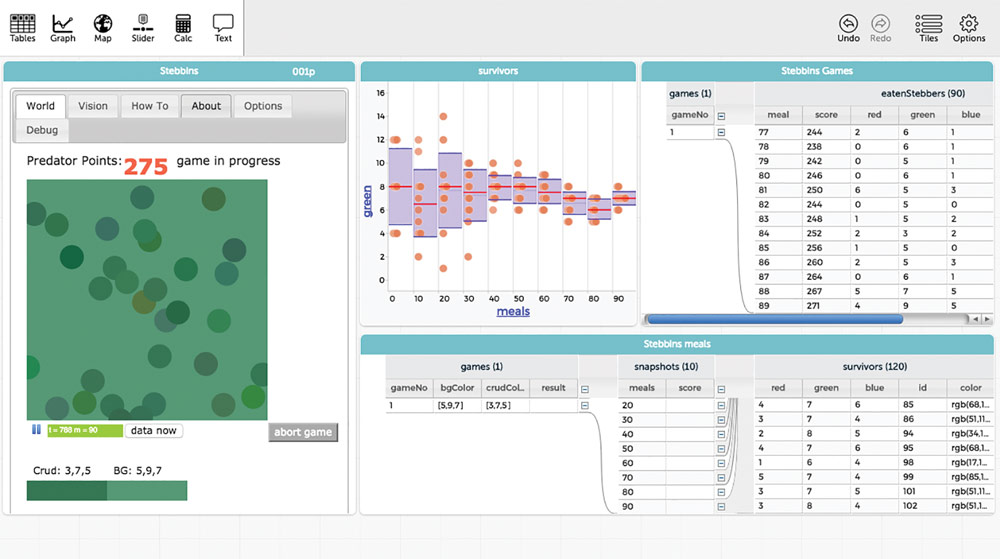
In August 2016, eight San Francisco Unified School District secondary science teachers tested game prototypes and lesson ideas and provided realistic perspectives on connections to classroom practice. Teachers offered feedback to improve each game and participated in a brainstorming session to design a chemistry data science game aligned to NGSS. One teacher said, “I definitely see myself using CODAP in my classroom to have students generate graphs and analyze data. I will be playing for a few hours when I get home!”
Six middle and high school teachers will teach a two-week curriculum unit focused on one or more multi-level games, in which students participate in game-playing episodes interspersed with classroom activities and discussion. Based on classroom testing and feedback, we will improve curriculum materials for each unit.
Research
Our research focuses on discovering ways this new genre can be integrated into classroom learning, and how data science games can be used to increase student encounters with data-rich situations. We expect to learn how students reason with data in ways we have not been able to before at the pre-college level. Specifically, we are interested in exploring young people’s conceptions of data structures and other data science competencies. Interviewing students as they interact with data science games will help us understand the learning processes and the challenges students experience as they work with data structures. Later, we will move toward a broader exploration of student learning and behavior in workshop and classroom settings to better understand the classroom supports and scaffolds required to encourage learning.
This research will inform the design and development of future data science educational tools and serve as the framework for guidelines that other educators can use to design and develop data science games. We hope to provide models of how to integrate learning data science into established content areas.
The future of data science
We are optimistic that data science games can create a path for students to learn how to make data-driven decisions in both games and life. We are excited about the potential of data science games and invite others to consider how to include data science across the curriculum—in games or other inventive ways.
Data science games can be developed by any software or curriculum developer and embedded easily in CODAP. Contact us at dsg@concord.org for more information or check out the CODAP help videos for instructions to embed an interactive.
Natalya St. Clair (nstclair@concord.org) is a research associate/project manager.
This material is based upon work supported by the National Science Foundation under grant IIS-1530578. Any opinions, findings, and conclusions or recommendations expressed in this material are those of the author(s) and do not necessarily reflect the views of the National Science Foundation.