Data and Analytics Spotlight
One of the Concord Consortium’s focus areas involves determining new ways to understand deep student learning of skills and processes such as science practices and engineering design. We’ve pioneered this research area for many years, incorporating data analytics into open-ended environments, simulations and tools. This past year, new employees and projects are redoubling our efforts.
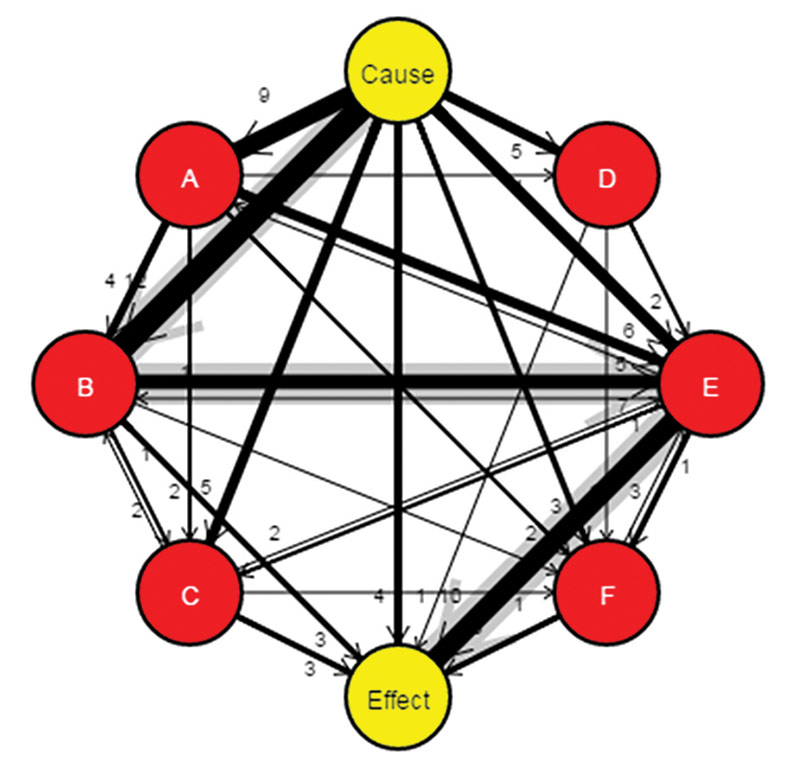
Dr. Jie Chao recently joined the Concord Consortium as a learning scientist with extensive research experience in technology-enhanced learning environments and STEM education. Dr. Chao completed her doctoral and postdoctoral training in instructional technology and STEM education at the University of Virginia. Her past research experiences range from fine-grained qualitative mental process analysis to large-scale quantitative and longitudinal investigations. She is currently focusing on learning analytics research in open-ended domains such as engineering design and authentic scientific inquiry. With insights in learning sciences and a strong, computationally oriented mindset, she hopes to utilize learning analytics to investigate important questions with unprecedented granularity and generate knowledge for technology and curriculum design that fosters student learning.
Dr. Chao and longtime Concord Consortium senior scientist Charles Xie are applying learning analytics in multiple ways to uncover new insights about essential processes in science and engineering. Dr. Xie is developing novel process analytics and concept map analytics that aim to probe into students’ learning of science and engineering concepts and skills through scientific inquiry and engineering design (Figure 1).
Dr. Hee-Sun Lee, another recent addition to our research staff, has spent the past two decades specializing in science education, assessment of curricula and development of technology-enhanced curricula. Dr. Lee received her M.S. in physics and Ph.D. in science education from the University of Michigan, where her thesis focused on science argumentation with middle school students. Since then, she has worked at the University of California at Berkeley, Tufts University and the University of California, Santa Cruz. Dr. Lee examines data from technology-based STEM learning environments.
In the InquirySpace project, Dr. Lee has used streamed logs of student interactions with a novel take on Bayesian Knowledge Tracing, a learning analytics technique for analyzing fine-grained data about student actions. Dr. Lee and project staff have identified and categorized students’ learning status in real time as they play a simplified game within our data exploration environment, CODAP. This work has uncovered new domains of understanding that can help guide later automatic assessment or scaffolding of students’ learning while they are in process (see “Analytics and Student Learning: An Example from InquirySpace,” page 8). Dr. Lee and other Concord Consortium staff are also using data from student actions to run natural language analysis of student arguments, develop real-time automatic feedback, and provide scaffolding supporting students as they explore Earth science models and simulations.
We’re pushing into new arenas of data analysis and learning analytics. Through this cutting-edge work, we hope to uncover new opportunities for rich assessment, timely and meaningful student and teacher feedback, and deepened teaching and learning.
Like Us, Follow Us
We’re thrilled to have you as friends and followers. Get the latest updates on happenings at the Concord Consortium by following us on these sites.